Listen to this Museletter
Welcome to the future of healthcare, where diseases are outsmarted before they even begin! In this edition of The Agna Museletter, we dive into how AI is revolutionising Life Sciences—from digital twins that track your biology in real-time to nanopills delivering pinpoint treatments. It’s not just innovation; it’s a healthcare reinvention.
Right now,
- AI is tackling the colossal 12–15 years and $2.5 billion it takes to bring a drug to market.
- Tools like AI-driven patient recruitment, 3D-printed personalised implants, and predictive diagnostics are accelerating breakthroughs while slashing inefficiencies.
- With 14% success rates in clinical trials, AI is transforming failures into life-changing innovations, faster and smarter.
But challenges like biased datasets and fragmented systems remain. Governments and startups alike are rising to the occasion, building frameworks like the EU AI Act and data-sharing hubs to ensure AI reaches its full potential. Dive into this issue to explore how today’s decisions are shaping a future where healthcare doesn’t just treat—it anticipates.
Museletter Highlights:
- Agna Insights
Read through the transformative impact of AI in Life Sciences - Agna Perspectives
Interesting and insightful reads from our social media - On the ‘Front’ier Tech
Latest updates from the Frontier Tech Ecosystem - Agna Recommends
Team Agna’s curated recommendations for you
Agna Insights
Note: If you are an enthusiast, practitioner, investor, or an expert – you know that this is a pivotal moment to shape Life Sciences with AI-driven healthcare.
A Vision of the Future: AI Revolutionising Life Sciences
Picture this: it’s the year 2100. Healthcare has become predictive, personalised, and deeply proactive. Diseases like cancer and Alzheimer’s are not defeated in a traditional sense but are preempted entirely. These breakthroughs stem from the synergy between AI and innovations in Life Sciences.
In this future, individuals carry digital twins—AI-powered replicas of their unique biology. These digital companions monitor physiological changes in real-time, alerting both the individual and healthcare systems before any abnormality becomes a threat. Alongside this, nanopills, a product of advanced nanotechnology and AI, play a pivotal role. These microscopic pills travel through the body, delivering medication with laser-like precision while collecting and transmitting vital health data directly to AI systems. The result? A seamless loop of continuous monitoring and intervention tailored to each person’s unique needs.
The Present Impact: AI’s Potential Today
While 2100 may seem distant, the foundations of this future are being built today. AI is already tackling systemic inefficiencies across healthcare, from drug discovery to treatments.
Artificial Intelligence: AI In Drug Discovery
The Traditional Puzzle of Discovery: The Challenges in Drug Discovery to Clinical Trials
The journey of discovering and developing drugs or devices is akin to solving an intricate puzzle—a lock-and-key analogy that has defined the process for decades. The lock represents the complexity of diseases, each unique in its biological makeup, while the key symbolises the precise treatment required to address it. Traditionally, this journey begins in wet labs, where researchers test thousands of chemical compounds to see how they interact with biological targets, a process known as high-throughput screening. Imagine testing keys until one fits the lock—this is an enormous, time-consuming effort.
Why It’s So Hard: The Challenges of Traditional Approaches
The human body’s complexity is both fascinating and formidable. Drug discovery timelines average 12–15 years, with costs exceeding $2.5 billion per drug. Moreover, only about 9–14% of candidates that enter early-stage clinical trials (Phase I, focused on safety testing in small groups of people) achieve regulatory approval. This means that the vast majority of potential drugs fail at some stage, often due to unforeseen issues like side effects or a lack of effectiveness in patients.
This high failure rate stems from several factors. For one, our understanding of diseases—especially their molecular and genetic underpinnings—is still incomplete. Predicting how a drug will behave in the human body is difficult, as patient responses vary based on genetic, environmental, and lifestyle factors. For example, a treatment effective in one individual might fail in another due to differences in metabolism or immune responses.
Beyond these biological challenges, the process faces systemic hurdles. Clinical trials, which are designed to test drugs on human volunteers, require diverse patient populations to ensure the results apply to all demographics. Yet, recruiting patients can be slow and uneven, creating significant delays. Even after recruitment, data collection, patient monitoring, and trial management rely heavily on manual processes. These inefficiencies drive up costs and slow down the delivery of life-saving treatments.
Why This Matters
The challenges of drug and device discovery aren’t just scientific—they have real-world consequences. The long timelines and high costs contribute to rising healthcare expenses, making it harder for patients to access affordable treatment. Additionally, for rare diseases or conditions prevalent in underserved communities, the inefficiencies in the system mean that solutions are often delayed—or never developed at all.
The Key to all our Problems? – AI’s Role in Discovery and Clinical Trials
Discovery
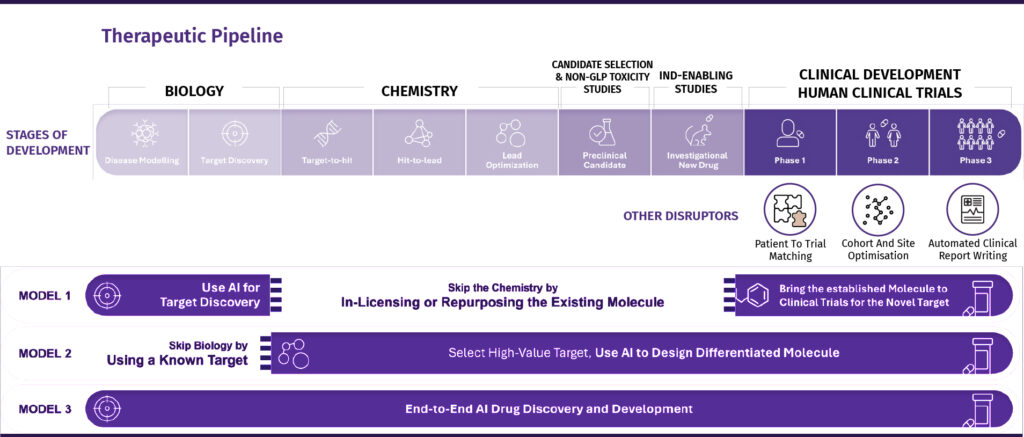
AI has showcased a lot of promise in this space, targeting the critical inefficiencies that we see today; let’s take examples,
- AI-Based Hypothesis Generation for Drug Repurposing or Licensing:
These companies leverage AI to identify potential disease mechanisms and therapeutic targets. They then acquire known drugs, repurpose generic medicines, or utilise readily available compounds to expedite the development process. This strategy allows them to bypass early-stage activities such as hit identification and optimisation, often enabling Phase II trials to commence rapidly. However, many such initiatives have failed to deliver positive outcomes, resulting in significant market devaluations. While this model reduces risks associated with drug chemistry, it carries substantial risks related to target selection. - Developing New Molecules for Established Targets:
Companies in this category focus on designing differentiated, high-performance molecules for well-validated, high-priority targets. By sidestepping the uncertainties of discovering and validating targets, they aim to innovate superior drug candidates through AI-driven design. The primary challenge lies in competing with well-resourced industry leaders, making chemistry innovation critical. This approach mitigates target selection risks but amplifies risks related to molecule design and chemistry. - Creating First-in-Class Molecules for Novel Targets:
These companies employ comprehensive AI platforms to identify unique, high-potential targets without direct clinical-stage competitors. They then design entirely new molecules to address these targets. An example of this model’s success is a Phase II study demonstrating both safety and efficacy. This approach involves elevated risks in selecting the right targets but offers moderate risks in chemistry and design due to the innovative focus.
Clinical and Human Trials
AI’s integration into clinical trials has opened avenues for addressing inefficiencies that traditionally delay or complicate the process. From patient recruitment to data analysis, several startups in the last few years have brought innovative solutions to market, although challenges remain.
- AI-Driven Patient Recruitment
One of the most critical bottlenecks in clinical trials is identifying and enrolling eligible patients. Startups like Deep 6 AI and Unlearn.AI are transforming this space. Deep 6 AI leverages natural language processing (NLP) and machine learning to mine electronic health records (EHRs) and rapidly identify trial-eligible patients. Unlearn.AI uses “digital twin” technology to simulate control groups for clinical trials, reducing the number of participants needed and expediting recruitment.
While these innovations have shown the potential to reduce recruitment timelines, they are not without challenges. EHR datasets are often incomplete or inconsistent, leading to gaps in AI-driven patient matching. Additionally, the adoption of these tools can vary geographically due to disparities in data availability and healthcare infrastructure.
- Monitoring and Managing Trials
Startups like Medable and Science 37 are enabling decentralised trial platforms that integrate AI-driven tools with wearable devices and remote monitoring systems. These solutions provide real-time insights into patient adherence, adverse events, and therapeutic outcomes. Decentralisation has gained traction, particularly in response to the logistical constraints imposed by the pandemic, allowing patients greater flexibility to participate from home.
Despite these advancements, decentralised trials bring their own complexities. Ensuring reliable connectivity and providing patients with user-friendly devices are logistical hurdles. Moreover, the sheer volume of data collected through continuous monitoring demands advanced analytics capabilities and raises privacy concerns.
- Data Analysis and Trial Outcomes
AI’s ability to process vast amounts of data has been a game-changer for trial analytics. Startups like Tempus and Owkin are making significant strides in this area. Tempus applies AI to analyse clinical and molecular data, providing actionable insights that accelerate trial decision-making. Owkin focuses on federated learning, enabling the training of AI models on distributed datasets without compromising data privacy—an essential feature in today’s regulatory environment.
However, the accuracy of AI’s analysis still depends heavily on the quality and diversity of the data. Biases in datasets can skew results, potentially compromising the reliability of conclusions drawn from these tools.
Manufacturing: From Implants to Devices – A New Era of Localised and Personalised Production
The evolution of manufacturing in MedTech is transforming how implants and diagnostic devices are designed, produced, and delivered. Traditionally, patients requiring implants like knees, hips, or jaws had to rely on standardised options—small, medium, or large—off-the-shelf solutions that often led to suboptimal outcomes, additional surgeries, and extended recovery times.
3D Printing: Personalised Implants and Bioresorbable Innovations
AI-powered 3D printing is addressing these challenges by enabling the creation of patient-specific implants tailored to individual anatomies. Companies like Axial3D are at the forefront, utilising AI-driven, cloud-based platforms to convert medical imaging data into accurate 3D models, facilitating enhanced surgical planning and improved patient outcomes.
A significant advancement in this field is the development of bioresorbable implants—temporary structures designed to be absorbed by the body over time, eliminating the need for secondary surgeries to remove them. Bioretec is pioneering this approach, focusing on creating strong, safe, and reliable bioresorbable implants for pediatric and adult orthopaedics.
Institutions like the Mayo Clinic are also adopting these innovations, establishing in-house 3D printing labs to address supply chain challenges and accelerate delivery. This shift is laying the groundwork for personalised implants to become a standard of care globally.
Healthcare Delivery: Expanding Access, Personalising Care, and Improving Efficiency
AI’s integration into healthcare delivery is revolutionising how patients are diagnosed, treated, and managed. From diagnostics to surgery, treatment management, and remote care, AI is enabling innovative solutions that address long-standing challenges in healthcare systems worldwide.
Diagnostics: Laying the Foundation for Personalised Care
Diagnostics serves as the first critical step in healthcare delivery, where accurate and early identification of diseases can significantly improve outcomes. AI has brought transformative advancements to this stage:
- Predictive Diagnostics: AI algorithms trained on vast datasets can identify disease patterns that are often imperceptible to human clinicians. For example, tools developed by PathAI analyse medical images to identify patterns, anomalies, and disease markers in tissue samples.
- AI-Driven Blood Tests: enabling the detection of early-stage cancers through simple blood tests, offering a less invasive diagnostic alternative.
- Personalised Risk Profiling: Personalised Risk Profiling: AI-driven solutions like Neko health analyse genetic, environmental, and lifestyle factors to create individualised risk profiles, empowering healthcare providers and patients to implement tailored preventive care strategies.
Surgery: Enhancing Precision and Efficiency
AI is transforming surgical care by enabling greater precision, automation, and improved patient outcomes:
- Surgical Robotics: Moon Surgical develops the Maestro System, a robotic surgical platform designed to assist surgeons with minimally invasive procedures, reducing recovery times and improving patient outcomes.
- Pre-Surgical Planning: AI-powered platforms such as those from Proprio generate detailed 3D models of patient anatomy, allowing surgeons to simulate and refine their procedures before entering the operating room.
- Intraoperative Guidance: Real-time AI tools such as Invenio Imaging guide surgeons during operations, enhancing accuracy and minimising errors. For instance, navigation systems ensure the precise positioning of surgical instruments during complex procedures.
Treatment Management: Personalising Care Beyond the Operating Room
After diagnosis and surgical intervention, ongoing care is essential for long-term recovery and quality of life. AI supports this phase by:
- Virtual Health Assistants: Virtual health assistant use cases are rapidly emerging across healthcare, offering support in areas such as administrative tasks, clinical workflows, and patient engagement. These AI-powered tools enhance efficiency by assisting with appointment scheduling, medication reminders, and chronic disease management. For instance, Suki helps streamline documentation for healthcare providers, allowing them to focus more on patient care.
- Remote Monitoring: AI-enabled devices such as AliveCor’s KardiaMobile continuously track vital signs, sending alerts to healthcare providers when abnormalities are detected. This ensures timely interventions and improves outcomes for patients managing chronic conditions.
- Dynamic Treatment Plans: Platforms like Tempus analyse real-time patient data to adjust therapies dynamically. This is especially critical in fields such as oncology, where treatment effectiveness can vary significantly between patients.
Addressing AI Barriers in Healthcare: Tackling Systemic and Technical Challenges
AI’s transformative potential in healthcare can only be realised through collaboration between governments, the private sector, and startups. However, progress is hindered by regulatory gaps, infrastructure limitations, and technical challenges such as data quality, algorithmic biases, and computational inefficiencies. Recognising these hurdles, governments are taking action to create enabling environments that facilitate AI adoption and drive meaningful impact.
Governments: Establishing Frameworks and Infrastructure
- Unified Data Sharing:
- Governments are investing in frameworks to facilitate secure and standardised data sharing:
- European Health Data Space (EHDS): Proposed to enable cross-border health data exchange, ensuring researchers and AI systems can access high-quality datasets securely. (European Commission)
- 21st Century Cures Act (US): Enforces interoperability rules to prevent information blocking and enable seamless exchange of patient health data. (ONC Health IT)
- These initiatives aim to eliminate data silos, a significant hurdle for training AI models effectively.
- Governments are investing in frameworks to facilitate secure and standardised data sharing:
- AI Regulatory Adaptation:
- The EU Artificial Intelligence Act introduces risk-based frameworks for regulating AI systems, ensuring they are safe, transparent, and non-discriminatory.
- Technical Capacity Building:
- Governments are funding AI research and skill development programmes. For instance:
- Singapore’s AI in Health Strategy emphasises training a dual-skilled workforce proficient in AI and healthcare.
- Governments are funding AI research and skill development programmes. For instance:
Technical Barriers: Challenges That Need Immediate Attention
- Data Quality and Diversity: Bias in datasets undermines AI accuracy, especially for diverse populations. Building representative, inclusive datasets requires global collaboration and shared accountability.
- Computational Limitations: Developing advanced AI demands significant computational power, often out of reach for smaller organizations. While quantum computing offers future promise, practical solutions are still emerging.
- Algorithmic Transparency: Black-box algorithms limit trust and adoption among clinicians. Explainable AI (XAI) technologies are in development but are not yet widely integrated into clinical practice.
- Integration into Clinical Workflows: AI tools often face resistance from healthcare providers due to disruptions in existing workflows. Intuitive design and effective clinician training programmes are essential to overcoming this barrier.
A Call to Action
The choices we make today—whether through investing in infrastructure & critical Life Science innovations, reforming regulations, or fostering collaboration—will shape the trajectory of healthcare for decades to come. This is our opportunity to build a system where diseases are detected before symptoms arise, treatments are designed with unparalleled precision, and healthcare reaches every corner of the globe.
The path to AI-powered healthcare is filled with challenges, but with the right focus, we can accelerate meaningful change. Addressing inefficiencies today will pave the way for a future of predictive, personalised, and accessible healthcare.
Agna Perspective
- Google DeepMind’s AlphaFold has revolutionised protein structure prediction, offering deeper insights into disease mechanisms. Read More
- Biomimicry —drawing inspiration from natural designs—offers practical solutions to healthcare’s toughest challenges. By combining biology with technology, scientists are creating scalable, sustainable tools, presenting an opportunity for investors to back transformative advancements. Read More
- Breast cancer diagnostics stand out, surging at 21.8% annually thanks to cutting-edge AI-driven screening. Read More
On the ‘Front’ier Tech:
- DeepSeek AI, a Chinese startup, has launched a free assistant that surpasses ChatGPT in downloads across multiple regions, including the US and UK. Known for its cost-efficiency and minimal data use, it faces concerns over censorship and data privacy.
- India’s first ‘Made in India’ chip is set to debut this year, as announced by Union Minister Ashwini Vaishnaw at the World Economic Forum. This marks a major milestone in India’s Rs 76,000 crore Semicon India programme, aimed at building a robust semiconductor manufacturing ecosystem.
Agna Recommends:
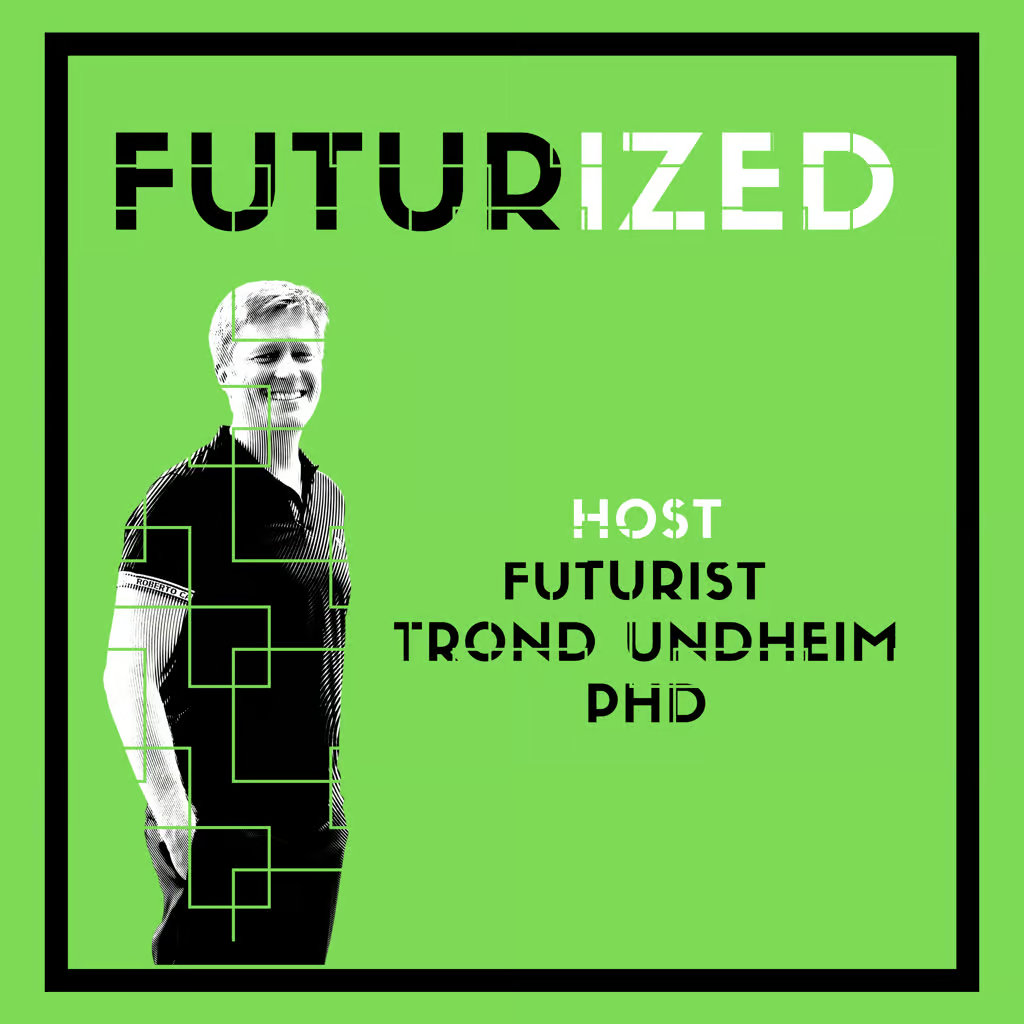
Futurized is a bi-weekly podcast by Trond Arne Undheim, exploring the societal impact of deep tech and preparing listeners for the disruptions of the next decade. Read More
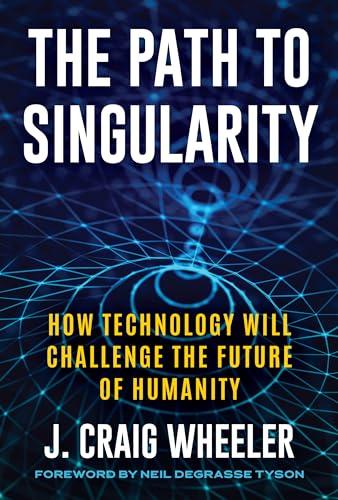
The Path to Singularity: How Technology Will Challenge the Future of Humanity by J. Craig Wheeler explores the concept of technological singularity, examining how rapid advancements in AI, robotics, and other emerging technologies may fundamentally alter human life and society. Read More
Listen to this Museletter
Questions? Feedback? Different perspective?
We invite you to engage with us and collaborate.
Warm Regards,
Team Agna
Click below to join our mailing list for The Agna Museletter.